In the world of prompt engineering, a pressing challenge has become increasingly evident: users often receive less-than-satisfactory responses due to poorly crafted prompt inputs. This issue is particularly significant in specialized applications like Sherlock AI, which is tailored for trading analysis. The problem arises due to users’ reliance on outdated search mental models. Trained by traditional search engines to rely on sparse keywords, users expect detailed and accurate outcomes from similar brief prompt inputs, which invariably fall short of their specific needs. Recognizing the limitations posed by outdated search mental models, we at Sherlock AI developed Auto-Prompt, an innovative feature that refines user queries through intelligent analysis and contextual enhancement before processing. This tool not only increases the accuracy of responses but also educates users on crafting more effective prompts, significantly boosting their proficiency over time. With the development of Auto-Prompt, we’ve taken a major leap forward in overcoming the obstacles inherent in prompt engineering, paving the way for more intuitive and efficient user experiences across applications enabling users with Generative AI.
Background
Traditionally, search engines function like digital librarians within vast libraries, responding to direct queries by retrieving the most relevant documents based on keyword matching. This method heavily relies on the user’s ability to craft effective queries using specific keywords. It’s akin to navigating a book’s index, where terms directly guide you to relevant pages. While this approach is effective for straightforward information retrieval, it often struggles with understanding the context and semantic meaning of the user. It remains static, limited to the literal words entered, and offers limited flexibility in handling complex queries.
In contrast, Generative AI and prompt engineering leverage the latest advancements in artificial intelligence to transcend mere keyword matching. This innovative approach enables applications to engage more interactively with users, interpreting and responding to the intent behind their queries rather than just the words themselves. In today’s data-driven decision-making environments, where the speed and accuracy of information retrieval are paramount, prompt engineering can play a crucial role. It not only enhances the user experience by providing more relevant and contextually aware responses but also transforms data interaction into a more intuitive and efficient process. By understanding the complexities and subtleties of user requests, prompt engineering helps bridge the gap between vast data repositories and meaningful outcomes.
The Limitations of Traditional Search Mental Models on Prompt Engineering
While prompt engineering significantly advances beyond traditional search methods, its implementation often encounters challenges when old mental models of search are applied. These traditional approaches, deeply ingrained in users’ habits, lead to several notable pitfalls in the realm of advanced prompt applications.
Persistence of Old Search Mental Models
Many users continue to interact with prompt engineering applications as they would with basic search engines—by inputting sparse keywords and expecting comprehensive outcomes. This habit stems from decades of conditioning by traditional search interfaces that prioritized simplicity and direct keyword relevance over contextual understanding. However, prompt engineering requires a more nuanced interaction, where the quality of the output is heavily dependent on the richness and precision of the input. Below, we will expand further and explore an example of a conventional query submitted to a search engine like Google, and demonstrate how such approaches can yield less-than-optimal results in a generative AI application such as Sherlock.
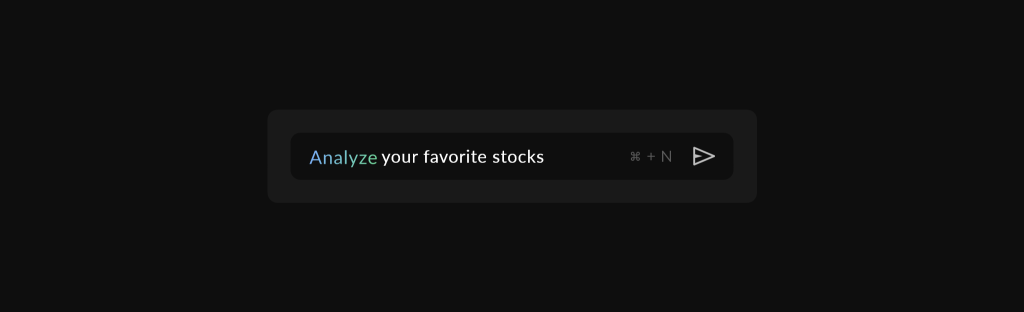
Inadequate User Inputs Leading to Suboptimal Outputs
The effectiveness of prompt engineering hinges on the user’s ability to articulate queries that are detailed and context-rich. Unfortunately, when users apply old search mental models, their inputs often lack the necessary detail, resulting in outputs that are either too broad or misaligned with the user’s actual needs. This discrepancy can lead to frustration, as users find themselves repeatedly refining their queries to achieve desired results, undermining the efficiency that prompt engineering is supposed to enhance. For instance, a query to Sherlock like “Does the valuation of PayPal make sense?” typically yields a broad response, offering little actionable value to the user.
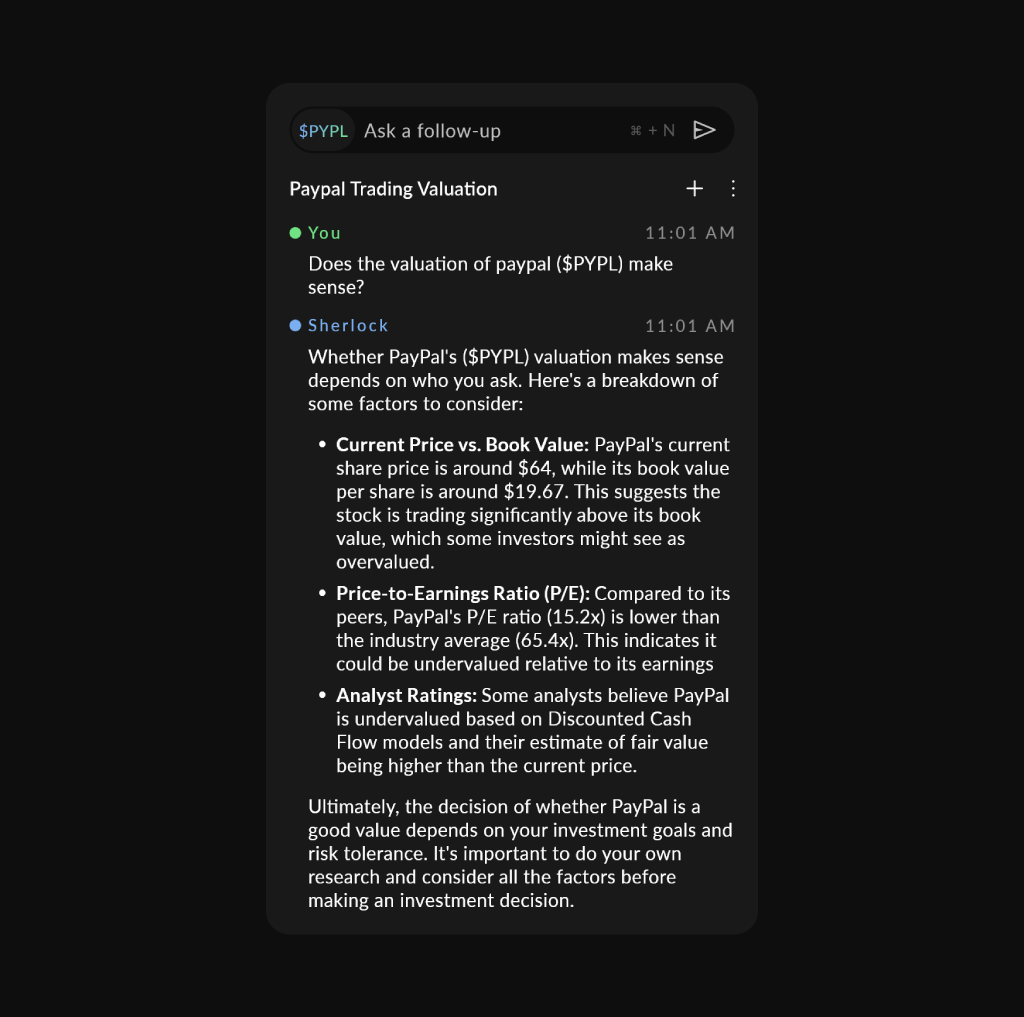
Impact on Sherlock’s Users
These challenges significantly affect the user experience while using our application, Sherlock AI, a trading co-pilot created to give retail traders access to advanced market analysis and help them manage risk. Persisting habits of entering sparse keywords, as if interacting with basic search engines, severely compromise the sophistication needed in prompt engineering for market analysis. When Sherlock AI users input vague or overly broad queries, the system’s advanced algorithms, designed to provide nuanced and detailed financial insights, sometimes yield substandard or misaligned information. This not only frustrates users, who must then repeatedly refine their inputs but also jeopardizes the efficiency and trust of our product.
Recognizing these challenges, our team at Sherlock AI was determined to create a solution that not only addresses these pitfalls but also redefines the user experience in AI prompt engineering.
The Genesis of Auto-Prompt
Understanding the critical need for improved prompt engineering in the trading and investing domain, we at Sherlock AI embarked on developing a groundbreaking feature: Auto-Prompt. This innovative tool is akin to auto-suggest but is tailored specifically for the unique challenges of prompt engineering. Auto-Prompt intelligently analyzes a user’s initial query, enhancing it by selecting appropriate data sources and adding necessary context before it reaches the LLM for processing. This pre-refinement process ensures that the queries are not only precise but also contextually enriched, leading to more accurate and actionable responses.
The Birth of an Idea
The idea for Auto-Prompt was born from a simple observation: many users struggle to craft effective prompts, often leading to frustrating experiences and suboptimal outcomes. Determined to solve this, I proposed a solution that would automatically refine user prompts to meet the high standards required for our AI to deliver its best performance. Excitedly, I shared this concept with my co-founder, envisioning a tool that would transform user interactions with our application. However, my initial enthusiasm was met with skepticism. My co-founder was quick to point out the limitations of current LLMs, suggesting that such a feature might not be feasible given the existing technological constraints.
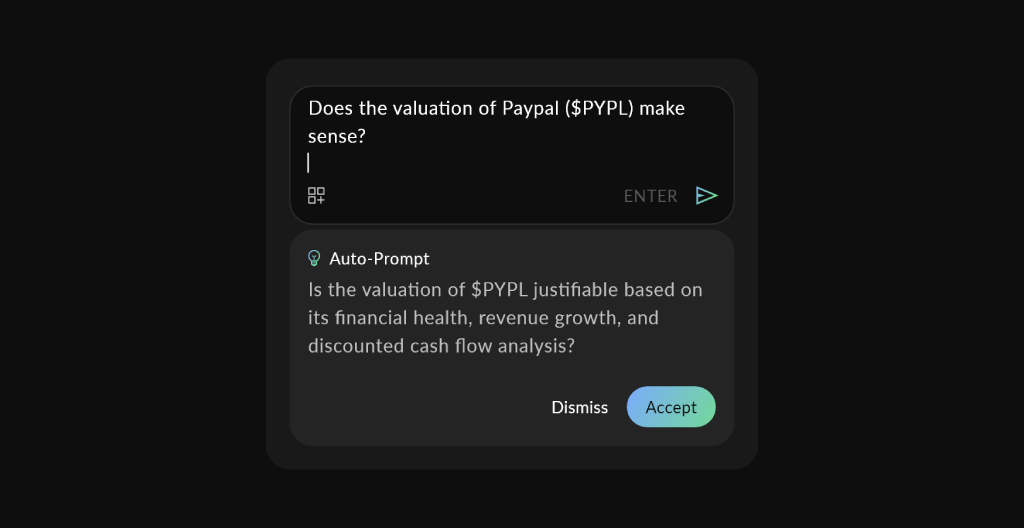
A Turnaround Moment
Refusing to let the idea go, we revisited the problem, searching for a way to make it work. That’s when my co-founder recalled a recent video featuring Groq’s platform, known for its lightning-fast inference speeds and cost-efficiency. Intrigued by the possibility, we decided to run a real-time experiment using Groq’s technology to see if our concept could hold water. The results were nothing short of astounding. Not only did the query refinement process work, but it also delivered results with remarkable speed and accuracy.
Refining the Idea
Lifted by this success, we set to work refining Auto-Prompt. Our goal was not only to improve response quality but also to educate our users on how to construct effective prompts. Each interaction with Auto-Prompt serves as a mini-tutorial, showing users the difference between a basic prompt and a well-constructed one, thus gradually enhancing their ability to use our system effectively. This feature not only empowers our users with better information but also trains them in the art of prompt engineering, making them more proficient and confident in their prompting abilities. As demonstrated by our example, the query refined by Auto-Prompt yielded a substantially more insightful and useful response for our user.
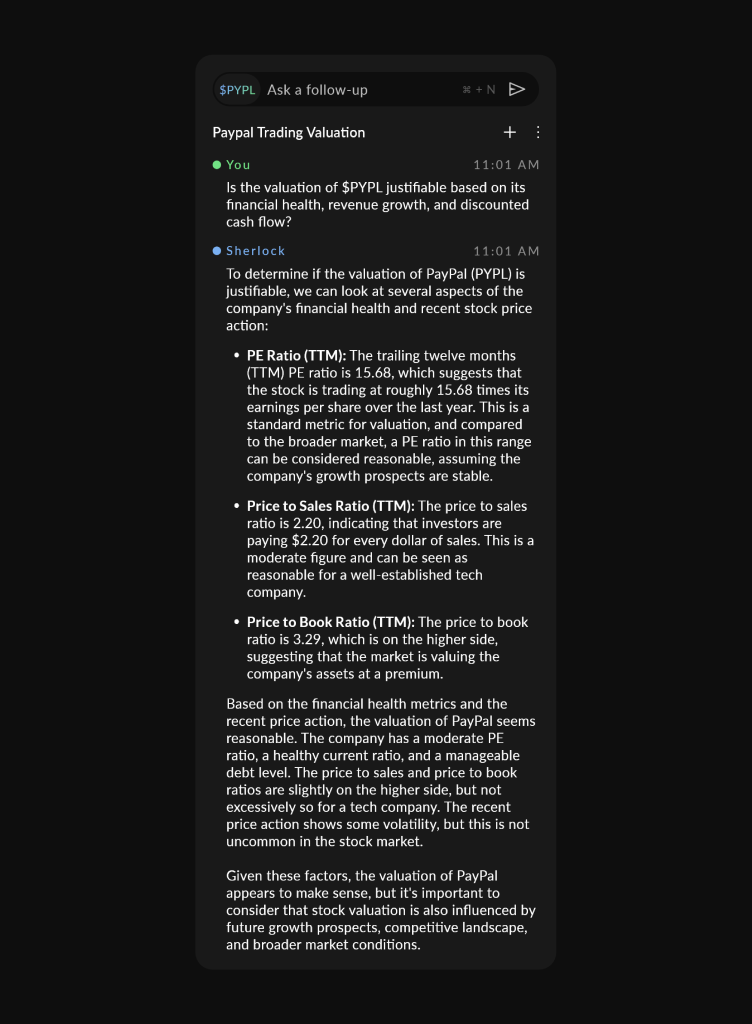
Conclusion
The journey of creating a transformative Generative AI product in trading has been marked with significant challenges, most notably the ability to give users contextually rich information given sparse prompt inputs. With the introduction of Auto-Prompt, Sherlock AI has not only addressed these challenges but has also set a new standard for interaction within Generative AI applications. By refining queries before they are processed, Auto-Prompt ensures that users receive highly relevant and contextually appropriate responses, thereby enhancing the decision-making process for traders. This advancement is not just a leap forward for Sherlock AI but a beacon for the entire industry, demonstrating the potential to significantly enhance Generative AI user experiences. As we continue to refine our product and expand its capabilities, we invite traders and investors to join us in redefining the future of market analysis, ensuring that they are equipped with the best tools to succeed in an increasingly competitive environment.